One platform.
Powerful results.
Waystar’s cloud-based software empowers healthcare organizations of all types and sizes with revenue cycle solutions to achieve increased performance, operational efficiency, cost reduction, and strategic growth.
Explore our platform
50%
of U.S. patient population
74+
provider net promoter score*
1M+
providers
*Third Party Provider Satisfaction Survey, September 2023
The Waystar Platform
Access everything you need in one place for end-to-end revenue cycle optimization. Discover why providers trust Waystar’s healthcare payments software to gain better financial visibility, speed up payments, increase revenue, improve productivity — and be successful from the start.
Financial Clearance
Verify insurance benefits, offer price transparency, automate prior authorizations, and more
Patient Financial Care
Offer self-service payment options, share personalized video EOBs, provide accurate estimates, and more.
Claim + Payer Payment Management
Automate claim monitoring, streamline attachments, manage payer remittances, and more.
Denial Prevention + Recovery
Avoid, track, and appeal denials to get paid faster and more fully
Analytics + Reporting
Get actionable intelligence from your data and generate easy-to-understand reports
Let’s make healthcare payments more human
Waystar’s award-winning platform is designed to meet everyone — healthcare financial leaders, revenue cycle management teams, and patients — where they are with solutions customized for their needs. Our advanced automation enables providers to focus on high-value efforts and put the human touch where it matters most: toward caring for their patients and communities.
Waystar Results
*Third Party Provider Satisfaction Survey, September 2023
Industry excellence











True North Client Conference
Unlock the potential of your Waystar solutions + think past the horizon in healthcare payments.
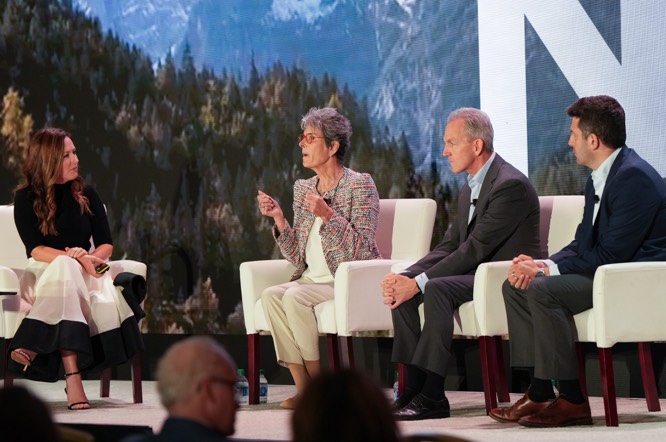
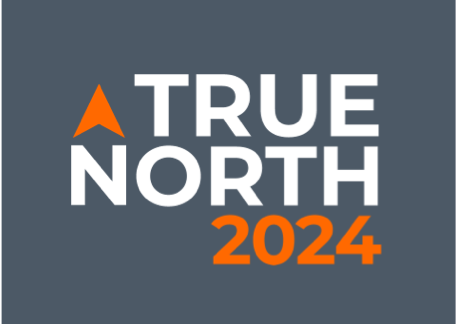
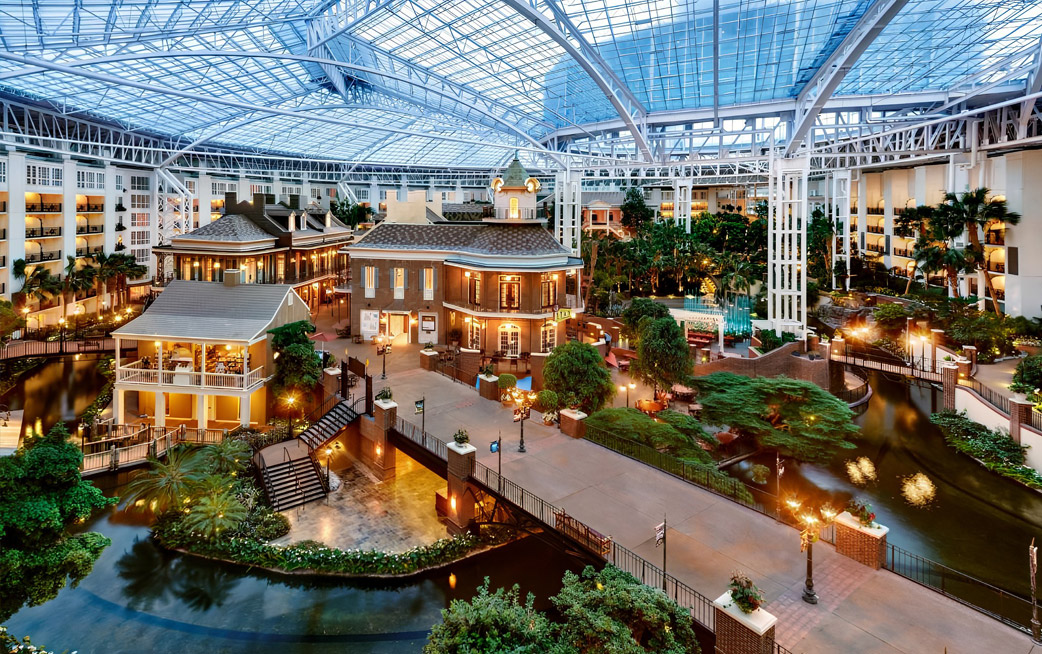
September 9-11
Disney’s Yacht & Beach Club Resort
Trending resources
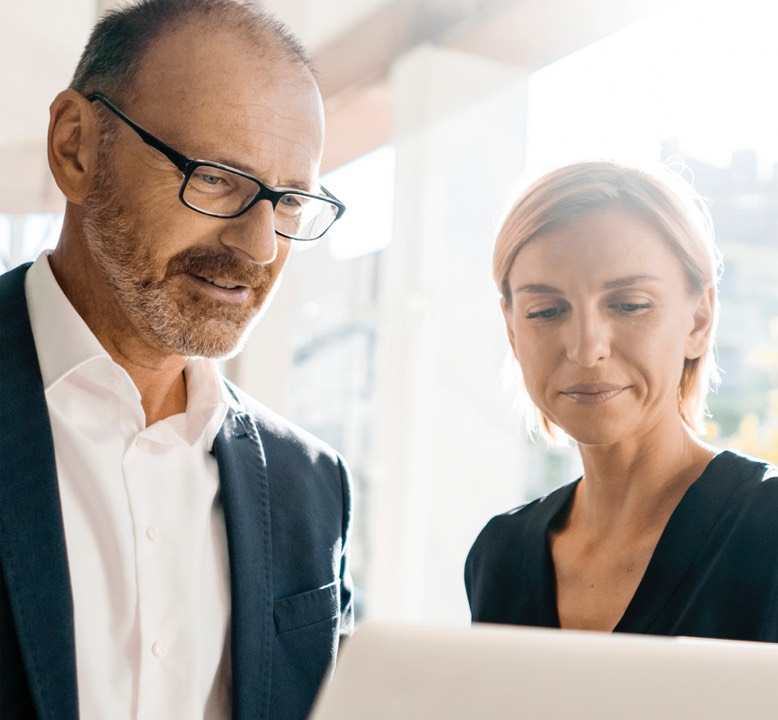
Outsmart denials with purpose-built technology
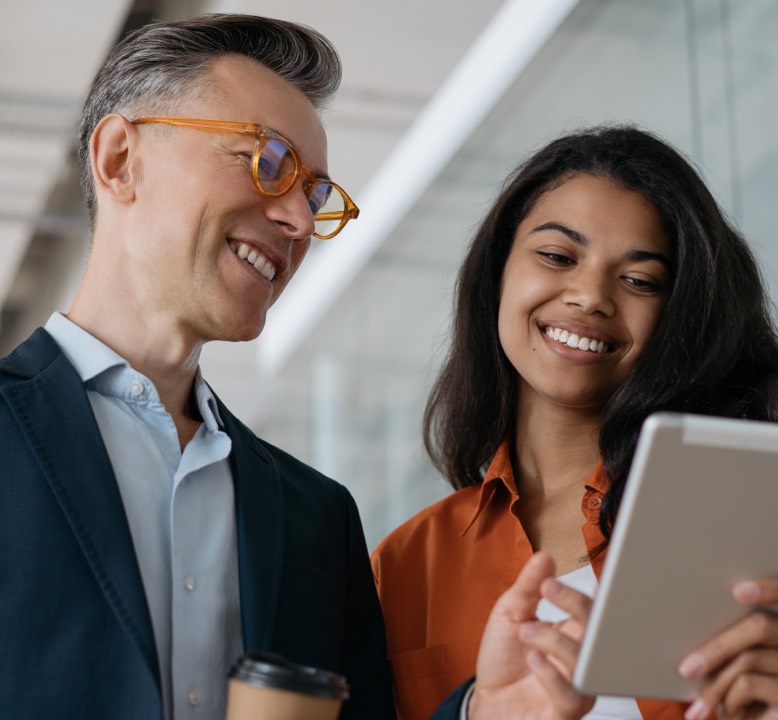
Piedmont’s path to platform integration
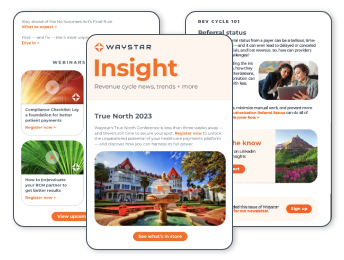